InChI Tag: Publication
Test (All Tags)
Can an InChI for Nano Address the Need for a Simplified Representation of Complex Nanomaterials across Experimental and Nanoinformatics Studies?
Nanomaterials 2020, 10(12), 2493; https://doi.org/10.3390/nano10122493
by Iseult Lynch, Antreas Afantitis, Thomas Exner, Martin Himly, Vladimir Lobaskin, Philip Doganis, Dieter Maier, Natasha Sanabria, Anastasios G. Papadiamantis, Anna Rybinska-Fryca, Maciej Gromelski, Tomasz Puzyn, Egon Willighagen, Blair D. Johnston, Mary Gulumian, Marianne Matzke, Amaia Green Etxabe, Nathan Bossa, Angela Serra, Irene Liampa, Stacey Harper, Kaido Tämm, Alexander Jensen, Pekka Kohonen, Luke Slater, Andreas Tsoumanis, Dario Greco, David A. Winkler, Haralambos Sarimveis and Georgia Melagraki
A possible extension to the RInChI as a means of providing machine readable process data
Philipp-Maximilian Jacob, Tian Lan, Jonathan M. Goodman & Alexei A. Lapkin
Journal of Cheminformatics volume 9, Article number: 23 (2017)
Abstract: The algorithmic, large-scale use and analysis of reaction databases such as Reaxys is currently hindered by the absence of widely adopted standards for publishing reaction data in machine readable formats. Crucial data such as yields of all products or stoichiometry are frequently not explicitly stated in the published papers and, hence, not reported in the database entry for those reactions, limiting their usefulness for algorithmic analysis. This paper presents a possible extension to the IUPAC RInChI standard via an auxiliary layer, termed ProcAuxInfo, which is a standardised, extensible form in which to report certain key reaction parameters such as declaration of all products and reactants as well as auxiliaries known in the reaction, reaction stoichiometry, amounts of substances used, conversion, yield and operating conditions. The standard is demonstrated via creation of the RInChI including the ProcAuxInfo layer based on three published reactions and demonstrates accurate data recoverability via reverse translation of the created strings. Implementation of this or another method of reporting process data by the publishing community would ensure that databases, such as Reaxys, would be able to abstract crucial data for big data analysis of their contents.
Analysing a billion reactions with the RInChI
Jonathan M. Goodman, Gerd Blanke and Hans Kraut
From the journal Pure and Applied Chemistry
Abstract: The RInChI is a canonical identifier for reactions which is widely used in reaction databases. It can be used to handle large collections of reactions and to link information from diverse data sources. How much information can it handle? Studies of the SAVI database, which contains more than a billion reactions, demonstrate that the RInChI is useful in analysing such a large collection of molecular data, and the reduced form of the Web-RInChIKey contains enough information to be an effective differentiator of reactions. Issues of NH tautomerism and stereochemistry are handled effectively. The RInChI illustrates that some of the properties of the algorithmically-generated SAVI database differ from SPRESI, which is a collection of experimental data. The RInChI has different properties to Reaction SMILES and both approaches provide useful and distinct information. We recommend that the RInChI be included in data models for reactions.
International chemical identifier for reactions (RInChI)
Guenter Grethe, Jonathan M Goodman & Chad HG Allen
Journal of Cheminformatics volume 5, Article number: 45 (2013)
Abstract: The IUPAC International Chemical Identifier (InChI) provides a method to generate a unique text descriptor of molecular structures. Building on this work, we report a process to generate a unique text descriptor for reactions, RInChI. By carefully selecting the information that is included and by ordering the data carefully, different scientists studying the same reaction should produce the same RInChI. If differences arise, these are most likely the minor layers of the InChI, and so may be readily handled. RInChI provides a concise description of the key data in a chemical reaction, and will help enable the rapid searching and analysis of reaction databases.
Enumeration of Ring–Chain Tautomers Based on SMIRKS Rules
Laura Guasch, Markus Sitzmann, and Marc C. Nicklaus
https://www.ncbi.nlm.nih.gov/pmc/articles/PMC4170818/
J Chem Inf Model. 2014 Sep 22; 54(9): 2423–2432.
Abstract: A compound exhibits (prototropic) tautomerism if it can be represented by two or more structures that are related by a formal intramolecular movement of a hydrogen atom from one heavy atom position to another. When the movement of the proton is accompanied by the opening or closing of a ring it is called ring–chain tautomerism. This type of tautomerism is well observed in carbohydrates, but it also occurs in other molecules such as warfarin. In this work, we present an approach that allows for the generation of all ring–chain tautomers of a given chemical structure. Based on Baldwin’s Rules estimating the likelihood of ring closure reactions to occur, we have defined a set of transform rules covering the majority of ring–chain tautomerism cases. The rules automatically detect substructures in a given compound that can undergo a ring–chain tautomeric transformation. Each transformation is encoded in SMIRKS line notation. All work was implemented in the chemoinformatics toolkit CACTVS. We report on the application of our ring–chain tautomerism rules to a large database of commercially available screening samples in order to identify ring–chain tautomers
Tautomerism of Warfarin: Combined Chemoinformatics, Quantum Chemical, and NMR Investigation
Laura Guasch, Megan L. Peach, and Marc C. Nicklaus
https://www.ncbi.nlm.nih.gov/pmc/articles/PMC7724503/
J Org Chem. 2015 Oct 16; 80(20): 9900–9909.
Abstract: Warfarin, an important anticoagulant drug, can exist in solution in 40 distinct tautomeric forms through both prototropic tautomerism and ring–chain tautomerism. We have investigated all warfarin tautomers with computational and NMR approaches. Relative energies calculated at the B3LYP/6-311G+ +(d,p) level of theory indicate that the 4-hydroxycoumarin cyclic hemiketal tautomer is the most stable tautomer in aqueous solution, followed by the 4-hydroxycoumarin open-chain tautomer. This is in agreement with our NMR experiments where the spectral assignments indicate that warfarin exists mainly as a mixture of cyclic hemiketal diastereomers, with an open-chain tautomer as a minor component. We present a diagram of the interconversion of warfarin created taking into account the calculated equilibrium constants (pKT) for all tautomeric reactions. These findings help with gaining further understanding of proton transfer and ring closure tautomerization processes. We also discuss the results in the context of chemoinformatics rules for handling tautomerism.
Experimental and Chemoinformatics Study of Tautomerism in a Database of Commercially Available Screening Samples
Laura Guasch, Waruna Yapamudiyansel, Megan L. Peach, James A. Kelley, Joseph J. Barchi, Jr., and Marc C. Nicklaus
https://www.ncbi.nlm.nih.gov/pmc/articles/PMC5129033/
J Chem Inf Model. 2016 Nov 28; 56(11): 2149–2161.
Abstract: We investigated how many cases of the same chemical sold as different products (at possibly different prices) occurred in a prototypical large aggregated database and simultaneously tested the tautomerism definitions in the chemoinformatics toolkit CACTVS. We applied the standard CACTVS tautomeric transforms plus a set of recently developed ring–chain transforms to the Aldrich Market Select (AMS) database of 6 million screening samples and building blocks. In 30 000 cases, two or more AMS products were found to be just different tautomeric forms of the same compound. We purchased and analyzed 166 such tautomer pairs and triplets by 1H and 13C NMR to determine whether the CACTVS transforms accurately predicted what is the same “stuff in the bottle”. Essentially all prototropic transforms with examples in the AMS were confirmed. Some of the ring–chain transforms were found to be too “aggressive”, i.e. to equate structures with one another that were different compounds
Tautomerism in large databases
Markus Sitzmann, Wolf-Dietrich Ihlenfeldt, and Marc C. Nicklaus
https://www.ncbi.nlm.nih.gov/pmc/articles/PMC2886898/
Journal of Computer-Aided Molecular Design volume 24, pages521–551 (2010)
https://link.springer.com/article/10.1007/s10822-010-9346-4#Ack1
Abstract: We have used the Chemical Structure DataBase (CSDB) of the NCI CADD Group, an aggregated collection of over 150 small-molecule databases totaling 103.5 million structure records, to conduct tautomerism analyses on one of the largest currently existing sets of real (i.e. not computer-generated) compounds. This analysis was carried out using calculable chemical structure identifiers developed by the NCI CADD Group, based on hash codes available in the chemoinformatics toolkit CACTVS and a newly developed scoring scheme to define a canonical tautomer for any encountered structure. CACTVS’s tautomerism definition, a set of 21 transform rules expressed in SMIRKS line notation, was used, which takes a comprehensive stance as to the possible types of tautomeric interconversion included. Tautomerism was found to be possible for more than 2/3 of the unique structures in the CSDB. A total of 680 million tautomers were calculated from, and including, the original structure records. Tautomerism overlap within the same individual database (i.e. at least one other entry was present that was really only a different tautomeric representation of the same compound) was found at an average rate of 0.3% of the original structure records, with values as high as nearly 2% for some of the databases in CSDB. Projected onto the set of unique structures (by FICuS identifier), this still occurred in about 1.5% of the cases. Tautomeric overlap across all constituent databases in CSDB was found for nearly 10% of the records in the collection.
Tautomer Database: A Comprehensive Resource for Tautomerism Analyses
Devendra K. Dhaked, Laura Guasch, and Marc C. Nicklaus
https://www.ncbi.nlm.nih.gov/pmc/articles/PMC8456363/
Abstract: We report a database of tautomeric structures that contains 2819 tautomeric tuples extracted from 171 publications. Each tautomeric entry has been annotated with experimental conditions reported in the respective publication, plus bibliographic details, structural identifiers (e.g., NCI/CADD identifiers FICTS, FICuS, uuuuu, and Standard InChI), and chemical information (e.g., SMILES, molecular weight). The majority of tautomeric tuples found were pairs; the remaining 10% were triples, quadruples, or quintuples, amounting to a total number of structures of 5977. The types of tautomerism were mainly prototropic tautomerism (79%), followed by ring–chain (13%) and valence tautomerism (8%). The experimental conditions reported in the publications included about 50 pure solvents and 9 solvent mixtures with 26 unique spectroscopic or nonspectroscopic methods. 1H and 13C NMR were the most frequently used methods. A total of 77 different tautomeric transform rules (SMIRKS) are covered by at least one example tuple in the database. This database is freely available as a spreadsheet at https://cactus.nci.nih.gov/download/tautomer/.
Toward a Comprehensive Treatment of Tautomerism in Chemoinformatics Including in InChI V2
Devendra K. Dhaked, Wolf-Dietrich Ihlenfeldt, Hitesh Patel, Victorien Delannée, and Marc C. Nicklaus*
https://www.ncbi.nlm.nih.gov/pmc/articles/PMC8459712/
Abstract: We have collected 86 different transforms of tautomeric interconversions. Out of those, 54 are for prototropic (non-ring–chain) tautomerism, 21 for ring–chain tautomerism, and 11 for valence tautomerism. The majority of these rules have been extracted from experimental literature. Twenty rules, covering the most well-known types of tautomerism such as keto–enol tautomerism, were taken from the default handling of tautomerism by the chemoinformatics toolkit CACTVS. The rules were analyzed against nine differerent databases totaling over 400 million (non-unique) structures as to their occurrence rates, mutual overlap in coverage, and recapitulation of the rules’ enumerated tautomer sets by InChI V.1.05, both in InChI’s Standard and a Nonstandard version with the increased tautomer-handling options 15T and KET turned on. These results and the background of this study are discussed in the context of the IUPAC InChI Project tasked with the redesign of handling of tautomerism for an InChI version 2. Applying the rules presented in this paper would approximately triple the number of compounds in typical small-molecule databases that would be affected by tautomeric interconversion by InChI V2. A web tool has been created to test these rules at https://cactus.nci.nih.gov/tautomerizer.
Andrew P. Cornell, Robert E. Belford
Chemistry Department, University of Arkansas at Little Rock, Little Rock, Arkansas 72204
Abstract
Many individual chemicals have a specific page on Wikipedia that will give information about the use, manufacture and properties of that chemical. The properties that are displayed off to the side include the relevant chemical identifiers along with alternate names and reaction information. There are several different identifier formats displayed within the properties box that include InChI (International Chemical Identifier), SMILES (Simplified Molecular-Input Line-Entry System) and various registration numbers. This lesson will explain how Python can be used to web scrape Wikipedia and retrieve the InChI after the user inputs a chemical name. Web scraping is a process for extracting the contents of a web page. This is often useful for working with online sources that do not offer an API (Application Programming Interface) for certain types of data. Wikipedia does have API’s for a lot of the information published, however this tutorial would like to look at the technique of web scraping with Python as an alternate method.
This program will work by importing a few helper modules that will allow the Python program to go onto the web, grab an HTML file and then parse the file specifically for the InChI string. Retrieving a valid result means that the user must input a chemical name that has a page designated on Wikipedia. Many chemicals have multiple names, so Wikipedia handles this through making the most commonly used name to be expressed in the URL (Uniform Resource Locator). All other naming formats will redirect to the URL that uses the chemicals common name.
Learning Objectives
- Import Python Library
- Create and Define Functions
- Parse HTML Text
- Display Results
Recommended Reading
- Internet of Chemistry Things Activity 1 (https://ioct.tech/edu/ioct1) Page that explains basic Instructions for setting up Python on a computer. The Python Activities listed in the sidebar may also help to explain some of the background information.
- Spring ChemInformatics OLCC Course (http://olcc.ccce.divched.org/Spring2017OLCC) This site provides lots of information on working with chemical data.
- Python Documentation (https://docs.python.org/3/) Python 3 documentation that correlates to the version used within this tutorial.
- Beautiful Soup Module (https://www.crummy.com/software/BeautifulSoup/bs4/doc/) Documentation on the installation and use of this module with Python.
Methods
The Python File used in this tutorial can be located within the following GitHub Page along with a DOI (Digital Object Identifier) on FigShare.1 Python will run on many different operating systems, however this tutorial uses the Thonny IDE (Integrated Development Environment) to design, run and test the code.2 The following code will take a chemical name and insert this into a preformatted URL that will pull all of the html from a corresponding Wikipedia page. The code will then parse and separate out everything in the html from the InChI identifier displaying the results.
Python 3 has been used for all code in this tutorial so make sure to consult the correct version documentation if additional reference is needed. Should the syntax or format change with future updates to the Python Language, it may be necessary to approach the task in a different way. The steps are broken down into sections which should be placed into the file one after the other from top to bottom.
Step 1
Starting with the libraries and modules that need to be declared, enter the code in step 1. The first line will import a function called “urlopen” from a library module called “urllib.request”. This will be responsible for allowing the program to fetch URL’s. The second line will import a library called “BeautifulSoup” from the package “bs4”. This module will be responsible for isolating the html text that we would like to retrieve as a result. The last module that will be imported is called “re” and this will be used to make some regular expressions that look for the pattern defined in the programs code which will contain the results.
The Python documentation recommended may be helpful with getting a deeper understanding of how importation of libraries into the program works. Be sure that the following code in step 1 is placed at the top of the file.
from urllib.request import urlopen from bs4 import BeautifulSoup import re
Step 2
After making the imports, add the following code which will define the first variable stored by Python. The name of the variable will be called “chemical” and it will store the value given through the text input displayed to the user. The variable stored should be a type of chemical identified by either its common name or systematic chemical name.
chemical = input("Put in the name of the chemical you want the InChI for: ")
Step 3
Two more variables will be necessary in setting up the preformatted URL structure needed to find corresponding chemical page on Wikipedia. The following code will set “html” as the variable and it will be assigned a full URL that is a combination of a preformatted section that does not change along with a piece that takes the user input defined in step 2. The URL will be pieced together into a single string matching where the chemical page is located on Wikipedia. In programming, this process is often called concatenation. The command “urlopen” will serve as the function or assignment to that page defining how to use the variable when called. After the “html” variable has been stored, a second variable called “wikiExtract” will store the text retrieved from this webpage. The first piece in the parenthesis will define what variable to call for the URL assignment followed by the format and the command for what should be done. The command “get_text” will then store everything on the page to the variable defined.
html = urlopen("https://en.wikipedia.org/wiki/" + chemical) wikiExtract = BeautifulSoup(html, "lxml").get_text()
Step 4
After the html of the webpage has been retrieved, the next few lines will search, isolate and store the InChI value independently of the other text from the webpage. The first line will perform a search of the html for the pattern “InChI=.*” and put this into memory as a value. The star and dot in the pattern will tell the program to grab everything found after “InChI=” as a parameter. Once the value has been found, the second line of code will then break off all text that follows the InChI string and store only the string to a new variable. The last function will provide the most refinement in isolating the InChI string by removing any added space or unwanted characters. The variable “inchiFinal” will be sent to the users display as the result of the search.
inchiMatch = re.findall("InChI=.*", wikiExtract) inchiClean = inchiMatch[0].split('H\\') inchiFinal = inchiClean[0].split()
Step 5
Before the user receives the results, the following code can be inserted to give a nice little message that is followed by the actual InChI string. This will help to keep things looking nice and clean.
print("\n" + "Wikipedia says the InChI is:" + '\n') print(inchiFinal[0])
If you would like to just copy the entire program in sequence, below is the completed code containing everything that is needed to perform retrieving an InChI from Wikipedia.
Completed Code Example
from urllib.request import urlopen from bs4 import BeautifulSoup import re chemical = input("Put in the name of the chemical you want the InChI for: ") html = urlopen("https://en.wikipedia.org/wiki/" + chemical) wikiExtract = BeautifulSoup(html, "lxml").get_text() inchiMatch = re.findall("InChI=.*", wikiExtract) inchiClean = inchiMatch[0].split('H\\') inchiFinal = inchiClean[0].split() print("\n" + "Wikipedia says the InChI is:" + '\n') print(inchiFinal[0])
Program Demonstration
An interactive demo of this program is provided by Trinket in the online publication.5 The trinket can be visited at this location (https://trinket.io/python3/437c1f516a). The stored and printable copies will only contain screenshots below with descriptions as they cannot display the trinket program in a live environment.
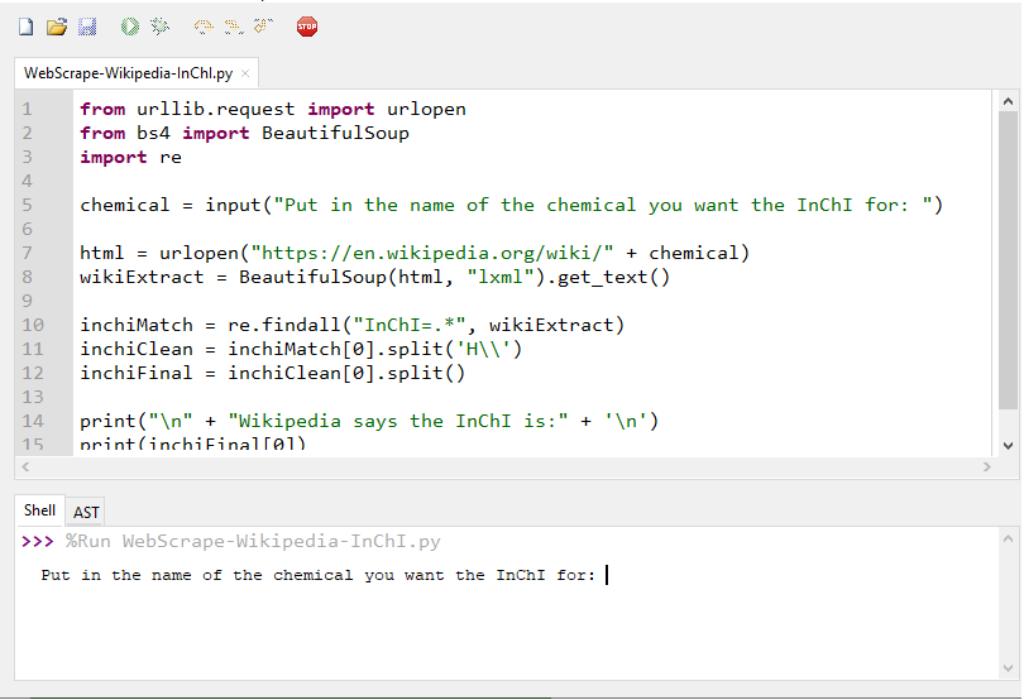
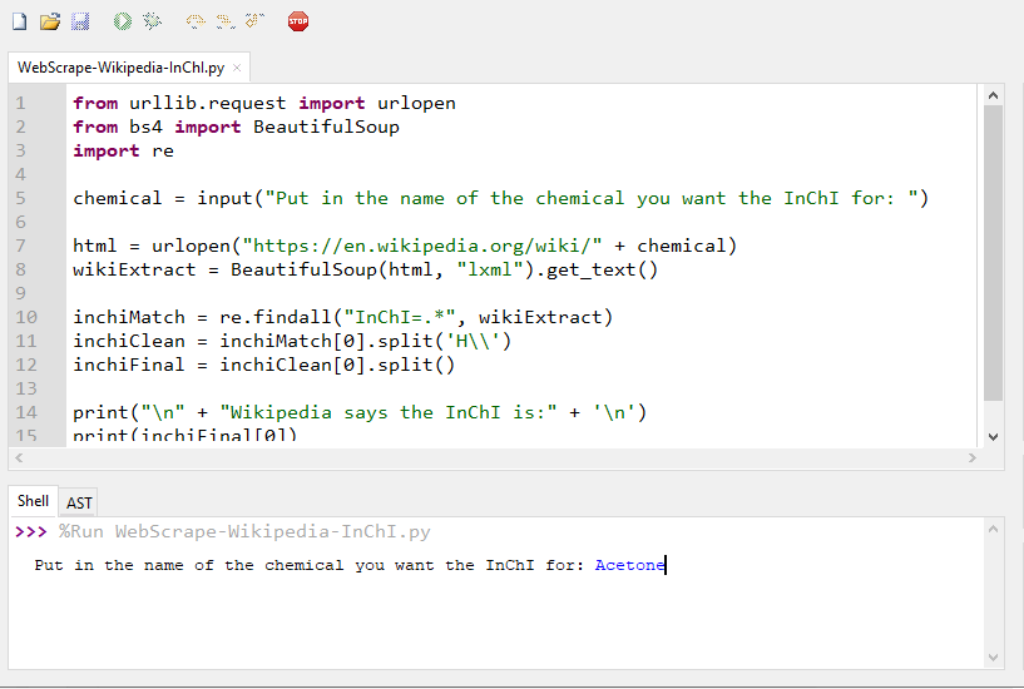
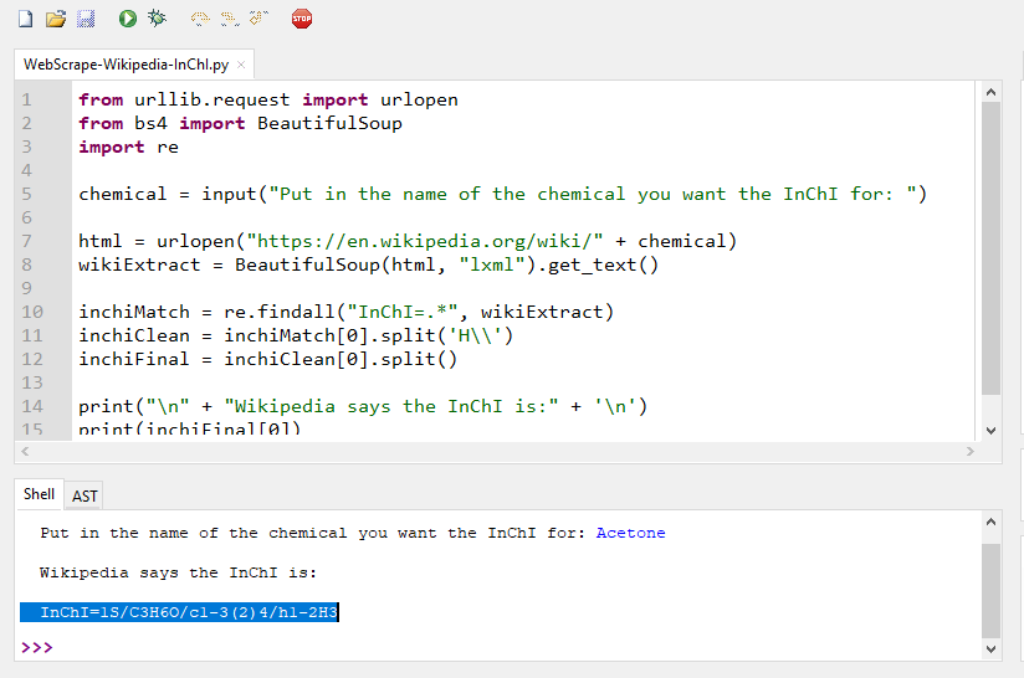
References
(1) | Cornell, A. Cheminformatics-Python. Figshare 2018. https://doi.org/10.6084/m9.figshare.7255901. |
(2) | Annamaa, A. Introducing Thonny, a Python IDE for Learning Programming. In Proceedings of the 15th Koli Calling Conference on Computing Education Research – Koli Calling ’15; ACM Press: Koli, Finland, 2015; pp 117–121. https://doi.org/10.1145/2828959.2828969. |
Andrew P. Cornell, Robert E. Belford
Chemistry Department, University of Arkansas at Little Rock, Little Rock, Arkansas 72204
Abstract
In this tutorial, a program written in Python will take a user specified chemical name and retrieve the associated chemical identifier or basic property using an online chemical database. This program can be used as both an aid for learning to programmatically work with chemical data and as a short general lesson for using Python with an API (Application Programming Interface). The database that will be used for this lesson is known as PubChem, which is a publicly accessible platform run by NCBI (National Center for Biotechnology Information).
PubChem offers public REST (Representational State Transfer) based programmatic access to a lot of the data contained on the servers which is defined with a specific syntax.1 Review the recommended reading to become familiar with the syntax if the need arises to pull data from PubChem that differs from this tutorial. This tutorial will use the InChI (International Chemical Identifier), InChI Key, molecular formula, Canonical SMILES (Simplified Molecular-Input Line-Entry System) and molecular weight with InChI being used in the demonstration section.2
Learning Objectives
- Import Python Library
- Create and Define Functions
- Make API Request with Python
- Parse and Display Results
Recommended Reading
- Internet of Chemistry Things Activity 1 (https://ioct.tech/edu/ioct1) Page that explains basic Instructions for setting up Python on a computer. The Python Activities listed in the sidebar may also help to explain some of the background information.
- Spring ChemInformatics OLCC Course (http://olcc.ccce.divched.org/Spring2017OLCC) This site provides lots of information on working with chemical data.
- Python Documentation (https://docs.python.org/3/) Python 3 documentation that correlates to the version used within this tutorial.
- PubChem REST Documentation (https://pubchemdocs.ncbi.nlm.nih.gov/pug-rest) Provides instructions on syntax structure, methods and request procedures for accessing data.
Methods
The files used in this tutorial can be located within the following GitHub Page (https://github.com/boots7458/Cheminformatics-Python) along with a DOI on FigShare (https://doi.org/10.6084/m9.figshare.7255901).3 Python will run on many different operating systems, however this tutorial will use the Thonny IDE (Integrated Development Environment) to design, run and test the code.4 The following code performs the commands that will retrieve either an InChI, InChI Key, molecular formula, SMILES or the molecular weight of a compound from PubChem. Each code section will be explained in detail with the full completed code located at the end of the tutorial. The completed code can be copied directly into a Python File and run as a fully functional program.
Python 3 has been used for all code in this tutorial so make sure to consult the correct version documentation if additional reference is needed. Should the syntax or format change with future updates to the Python Language, it may be necessary to approach the task in a different way. The steps are broken down into sections which should be placed into the file one after the other from top to bottom.
Step 1
When you run a python file it typically does not load user added modules. These modules are stored in specific libraries and are only loaded when programs need those features. So the first step will be to load a user requested library, the urllib.request – Extensible library for opening URLs (https://docs.python.org/3/library/urllib.request.html), which will define several functions and classes to help the program open URLs. This is done through the import command as shown in the following code.
import urllib.request
Step 2
After making the library import declaration, it is time to start defining a few functions that will build the different parts of the program. The first function is called “firstChoice” and will declare the “string2” variable as global. This will allow the variable to be called from any function without having to specifically pass it within the code. The function will be responsible for asking the user to input a text string that will be stored in memory as a variable for later use.
def firstChoice(): global string2 string2 = input("Enter a chemical name: ")
Step 3
The second function is much longer, despite performing a simple task. The function is called “choices” and it will ask the user to pick a number for which option to retrieve based on what the program is asking. The numbers inside the parenthesis next to the print statements define some formatting that will be displayed such as indents, line dividers and spacing for aesthetic purposes. The most important section is where “idChoice” is set as a global variable to store the value chosen by the user. The “if” and “elif” commands define what to do, depending on whether the user selects a valid number from the options or not. A valid option will simply pass the choice to be used in constructing the API (Application Programming Interface) URL for retrieving the user’s request. An incorrect option
will simply display a message stating the problem and to try again.
def choices(): print(40 * "_") print(3 * " " + "Select the value below to retrieve") print(40 * "_") print('{:>23}'.format("INCHI[0]")) print('{:>23}'.format("INCHIKEY[1]")) print('{:>23}'.format("MOLECULAR FORMULA[2]")) print('{:>23}'.format("SMILES[3]")) print('{:>23}'.format("MOLECULAR WEIGHT[4]")) print(40 * "_") global idChoice idChoice = int(input("Enter a number choice? ")) if 0 <= idChoice <= 4: choiceID() elif idChoice != range(0,4): print(2 * '\n' + 38 * '*') print("* Incorrect Number Choice, Try Again *") print(38 * '*' + 2 * '\n') choices()
Step 4
The next function in the program is called “choiceID” and this will take an array of values for the input and combine what the user has chosen into a string. The string will then be used for building the URL that will pull in the requested data value at the end. This is the function that will utilize the library from step 1 that was declared at the top of the file. The last part of the function will ask the user if another value should be requested or not. The program will end if the user types in “no” and will repeat the entire program if “yes” is entered as the user’s choice.
def choiceID(): inputList = ['INCHI', 'INCHIKEY', 'MolecularFormula','CanonicalSMILES', 'MolecularWeight'] selChoice = inputList[idChoice] string1 = "https://pubchem.ncbi.nlm.nih.gov/rest/pug/compound/name/" string3 = "/property/" string4 = "/TXT" html = urllib.request.urlopen(string1 + string2 + string3 + selChoice + string4).read() html2 = html.decode('UTF-8') print(2 * '\n') print(html2) print(2 * '\n') redoProg = input("Would you like to check another compound, yes or no? ") if redoProg == "yes": print ("\n" * 2) main() else: print("Done!")
Step 5
This function will be called “main” and it simply defines the order in which all other functions should be run. The program will run this first even though it is located towards the bottom of the Python File.
def main(): firstChoice() choices()
Step 6
Here is where the program is told which function to start with and it says to start with the function titled “main” which will define the order of which individual functions to run. This line of code is the starting point at which the program will read from after all libraries have been loaded. It is very important that this line of code take place after the functions along with being loaded after “Import Time” so that libraries are loaded before any code is executed. For this reason “main()” is written last in the program.
## Program Assignments ##
main()
Completed Code Example
import urllib.request def firstChoice(): global string2 string2 = input("Enter a chemical name: ") def choices(): print(40 * "_") print(3 * " " + "Select the value below to retrieve") print(40 * "_") print('{:>23}'.format("INCHI[0]")) print('{:>23}'.format("INCHIKEY[1]")) print('{:>23}'.format("MOLECULAR FORMULA[2]")) print('{:>23}'.format("SMILES[3]")) print('{:>23}'.format("MOLECULAR WEIGHT[4]")) print(40 * "_") global idChoice idChoice = int(input("Enter a number choice? ")) if 0 <= idChoice <= 4: choiceID() elif idChoice != range(0,4): print(2 * '\n' + 38 * '*') print("* Incorrect Number Choice, Try Again *") print(38 * '*' + 2 * '\n') choices() def choiceID(): inputList = ['INCHI', 'INCHIKEY', 'MolecularFormula','CanonicalSMILES', 'MolecularWeight'] selChoice = inputList[idChoice] string1 = "https://pubchem.ncbi.nlm.nih.gov/rest/pug/compound/name/" string3 = "/property/" string4 = "/TXT" html = urllib.request.urlopen(string1 + string2 + string3 + selChoice + string4).read() html2 = html.decode('UTF-8') print(2 * '\n') print(html2) print(2 * '\n') redoProg = input("Would you like to check another compound, yes or no? ") if redoProg == "yes": print ("\n" * 2) main() else: print("Done!") def main(): firstChoice() choices() ## Program Assignments ## main()
NOTES ON USING THE PROGRAM
This program will take a single chemical name without any modification needed. For example, if the user wants to look for data related to acetone, then the term can be entered as just “acetone”. However, if a user wants to enter a chemical name that contains more than one word, then a place marker must be put in so that the spaces can be accounted for in the URL Syntax. To enter the term acetic acid, the user should enter “acetic%20acid” by putting %20 anywhere that should contain a space, or the program will throw several errors as a result.
Program Demonstration
An interactive demo of this program is provided by Trinket in the online publication.5 The trinket can be visited at this location (https://trinket.io/python3/c845b6bdbd). The stored and printable copies will only contain screenshots below with descriptions as they cannot display the trinket program in a live environment.
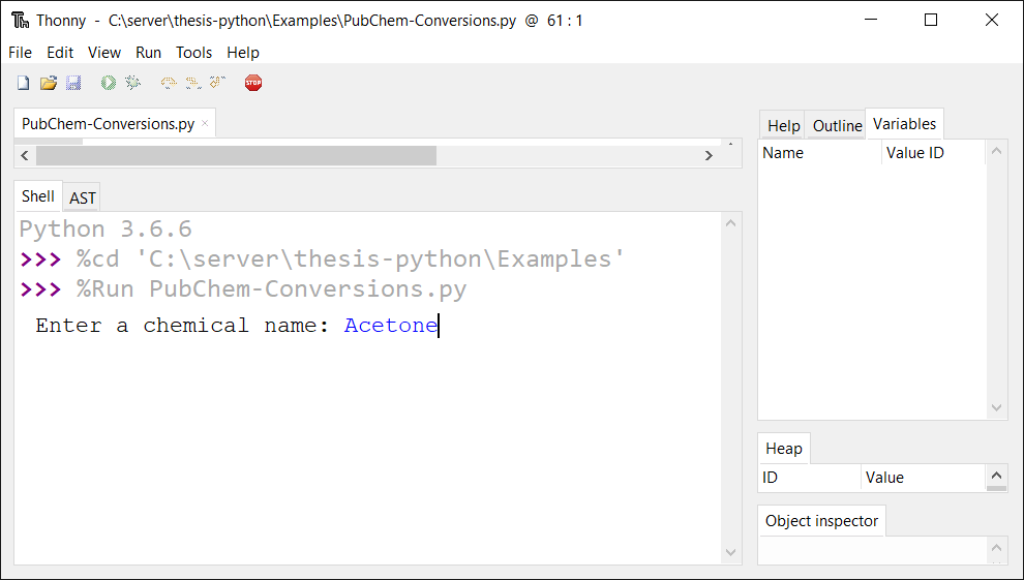
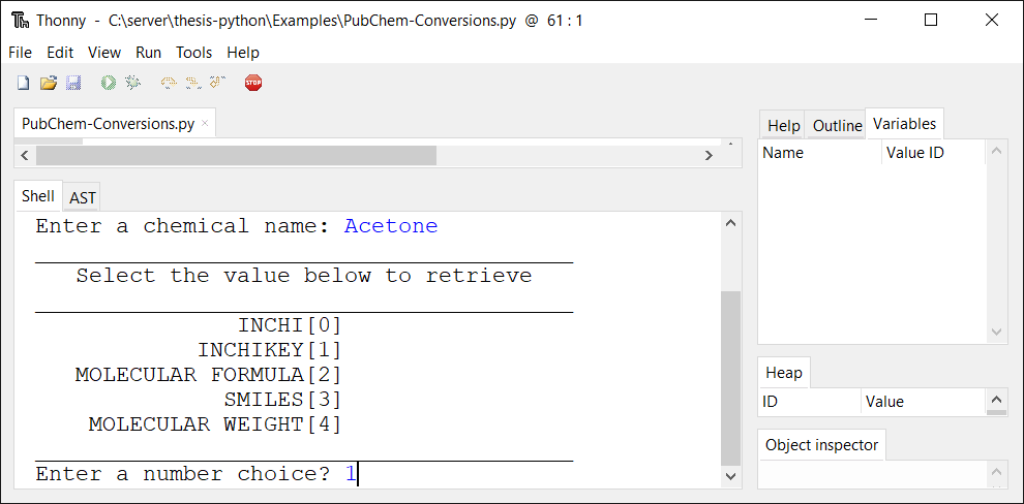
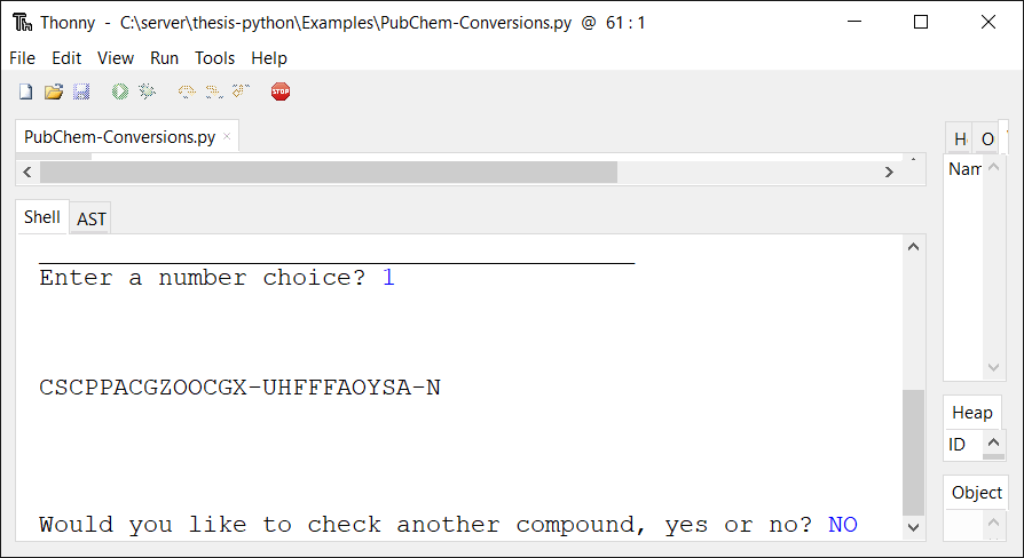
Suggested Questions for Classroom Use:
- What is a library in python, and why would python use libraries
- What library was used in the above code, and what does it do?
- What command allows you to make a function in python?
- What are the names of the functions the above program created?
- What does the command “global” do? Why is it needed?
- What is the URL that is generated to get the molar mass of aspirin?
- What does the code ‘{:>23}’ do?
- For the following code
idChoice = int(input("Enter a number choice? ")) if 0 <= idChoice <= 4: choiceID() elif idChoice != range(0,4): print(2 * '\n' + 38 * '*') print("* Incorrect Number Choice, Try Again *") print(38 * '*' + 2 * '\n') choices()
-
- (a) What does the following statements say?
if 0 <= idChoice <= 4: choiceID()
-
- (b) What does the following statements say? Can you think of another way of doing this?
idChoice != range(0,4):
-
- Go to the Compound Property Tables on this page https://pubchemdocs.ncbi.nlm.nih.gov/pug-rest$_Toc494865567
-
- Add a 6th option (option 5) that would allow you to get the IUPAC Name for a compound
- Add a 7th option (option 6) that would give you an indication if the compound is water or fat soluble.
References
(1) | Kim, S.; Thiessen, P. A.; Cheng, T.; Yu, B.; Bolton, E. E. An Update on PUG-REST: RESTful Interface for Programmatic Access to PubChem. Nucleic Acids Research 2018, 46 (W1), W563–W570. |
(2) | Heller, S. R.; McNaught, A.; Pletnev, I.; Stein, S.; Tchekhovskoi, D. InChI, the IUPAC International Chemical Identifier. Journal of Cheminformatics 2015, 7 (1). https://doi.org/10.1186/s13321-015-0068-4. |
(3) | Cornell, A. Cheminformatics-Python. Figshare 2018. https://doi.org/10.6084/m9.figshare.7255901. |
(4) | Annamaa, A. Introducing Thonny, a Python IDE for Learning Programming. In Proceedings of the 15th Koli Calling Conference on Computing Education Research – Koli Calling ’15; ACM Press: Koli, Finland, 2015; pp 117–121. https://doi.org/10.1145/2828959.2828969. |
(5) | Elliott Hauser; Brian Marks; Ben Wheeler. Trinket; 2019. |
Abstract
Background:
PubChem is a chemical information repository, consisting of three primary databases: Substance, Compound, and BioAssay. When individual data contributors submit chemical substance descriptions to substance, the unique chemical structures are extracted and stored into Compound through an automated process called structure standardization. The present study describes the PubChem standardization approaches and analyzes them for their success rates, reasons that cause structures to be rejected, and modifcations applied to structures during the standardization process. Furthermore, the PubChem standardization is compared to the structure normalization of the IUPAC International Chemical Identifer (InChI) software, as manifested by conversion of the InChI back into a chemical structure.
Abstract
Background:
Over the past several centuries, chemistry has permeated virtually every facet of human lifestyle, enriching fields as diverse as medicine, agriculture, manufacturing, warfare, and electronics, among numerous others. Unfortunately, application-specific, incompatible chemical information formats and representation strategies have emerged as a result of such diverse adoption of chemistry. Although a number of efforts have been dedicated to unifying the computational representation of chemical information, disparities between the various chemical databases still persist and stand in the way of cross-domain, interdisciplinary investigations. Through a common syntax and formal semantics, Semantic Web technology offers the ability to accurately represent, integrate, reason about and query across diverse chemical information.
Results:
Here we specify and implement the Chemical Entity Semantic Specification (CHESS) for the representation of polyatomic chemical entities, their substructures, bonds, atoms, and reactions using Semantic Web technologies. CHESS provides means to capture aspects of their corresponding chemical descriptors, connectivity, functional composition, and geometric structure while specifying mechanisms for data provenance. We demonstrate that using our readily extensible specification, it is possible to efficiently integrate multiple disparate chemical data sources, while retaining appropriate correspondence of chemical descriptors, with very little additional effort. We demonstrate the impact of some of our representational decisions on the performance of chemically-aware knowledgebase searching and rudimentary reaction candidate selection. Finally, we provide access to the tools necessary to carry out chemical entity encoding in CHESS, along with a sample knowledgebase.
Conclusions:
By harnessing the power of Semantic Web technologies with CHESS, it is possible to provide a means of facile cross-domain chemical knowledge integration with full preservation of data correspondence and provenance. Our representation builds on existing cheminformatics technologies and, by the virtue of RDF specification, remains flexible and amenable to application- and domain-specific annotations without compromising chemical data integration. We conclude that the adoption of a consistent and semantically-enabled chemical specification is imperative for surviving the coming chemical data deluge and supporting systems science research.
Abstract
Background:
The Blue Obelisk movement was established in 2005 as a response to the lack of Open Data, Open Standards and Open Source (ODOSOS) in chemistry. It aims to make it easier to carry out chemistry research by promoting interoperability between chemistry software, encouraging cooperation between Open Source developers, and developing community resources and Open Standards.
Results:
This contribution looks back on the work carried out by the Blue Obelisk in the past 5 years and surveys progress and remaining challenges in the areas of Open Data, Open Standards, and Open Source in chemistry.
Conclusions:
We show that the Blue Obelisk has been very successful in bringing together researchers and developers with common interests in ODOSOS, leading to development of many useful resources freely available to the chemistry community.
Abstract
UniChem is a freely available compound identifier mapping service on the internet, designed to optimize the efficiency with which structure-based hyperlinks may be built and maintained between chemistry-based resources. In the past, the creation and maintenance of such links at EMBL-EBI, where several chemistry-based resources exist, has required independent efforts by each of the separate teams. These efforts were complicated by the different data models, release schedules, and differing business rules for compound normalization and identifier nomenclature that exist across the organization. UniChem, a large-scale, non-redundant database of Standard InChIs with pointers between these structures and chemical identifiers from all the separate chemistry resources, was developed as a means of efficiently sharing the maintenance overhead of creating these links. Thus, for each source represented in UniChem, all links to and from all other sources are automatically calculated and immediately available for all to use. Updated mappings are immediately available upon loading of new data releases from the sources. Web services in UniChem provide users with a single simple automatable mechanism for maintaining all links from their resource to all other sources represented in UniChem. In addition, functionality to track changes in identifier usage allows users to monitor which identifiers are current, and which are obsolete. Lastly, UniChem has been deliberately designed to allow additional resources to be included with minimal effort. Indeed, the recent inclusion of data sources external to EMBL-EBI has provided a simple means of providing users with an even wider selection of resources with which to link to, all at no extra cost, while at the same time providing a simple mechanism for external resources to link to all EMBL-EBI chemistry resources.
Abstract
The Reaction InChI (RInChI) extends the idea of the InChI, which provides a unique descriptor of molecular structures, towards reactions. Prototype versions of the RInChI have been available since 2011. The frst ofcial release (RInChIV1.00), funded by the InChI Trust, is now available for download (https://www.inchi-trust.org/wp/downloads/). This release defnes the format and generates hashed representations (RInChIKeys) suitable for database and web operations. The RInChI provides a concise description of the key data in chemical processes, and facilitates the manipulation and analysis of reaction data.
Abstract
Background:
An important step in the reconstruction of a metabolic network is annotation of metabolites. Metabolites are generally annotated with various database or structure based identifiers. Metabolite annotations in metabolic reconstructions may be incorrect or incomplete and thus need to be updated prior to their use.
Genome-scale metabolic reconstructions generally include hundreds of metabolites. Manually updating annotations is therefore highly laborious. This prompted us to look for open-source software applications that could facilitate automatic updating of annotations by mapping between available metabolite identifiers. We identified three applications developed for the metabolomics and chemical informatics communities as potential solutions. The applications were MetMask, the Chemical Translation System, and UniChem. The first implements a “metabolite masking” strategy for mapping between identifiers whereas the latter two implement different versions of an InChI based strategy. Here we evaluated the suitability of these applications for the task of mapping between metabolite identifiers in genome-scale metabolic reconstructions. We applied the best suited application to updating identifiers in Recon 2, the latest reconstruction of human metabolism.
Results:
All three applications enabled partially automatic updating of metabolite identifiers, but significant manual effort was still required to fully update identifiers. We were able to reduce this manual effort by searching for new identifiers using multiple types of information about metabolites. When multiple types of information were combined, the Chemical Translation System enabled us to update over 3,500 metabolite identifiers in Recon 2. All but approximately 200 identifiers were updated automatically.
Conclusions:
We found that an InChI based application such as the Chemical Translation System was better suited to the task of mapping between metabolite identifiers in genome-scale metabolic reconstructions. We identified several features, however, that could be added to such an application in order to tailor it to this task.
Abstract
Background:
Correctness of structures and associated metadata within public and commercial chemical databases
greatly impacts drug discovery research activities such as quantitative structure–property relationships modelling and compound novelty checking. MOL files, SMILES notations, IUPAC names, and InChI strings are ubiquitous file formats and systematic identifiers for chemical structures. While interchangeable for many cheminformatics purposes there have been no studies on the inconsistency of these structure identifiers due to various approaches for data integration, including the use of different software and different rules for structure standardisation. We have investigated the consistency of systematic identifiers of small molecules within and between some of the commonly used chemical resources, with and without structure standardisation.
Results:
The consistency between systematic chemical identifiers and their corresponding MOL representation varies greatly between data sources (37.2%-98.5%). We observed the lowest overall consistency for MOL-IUPAC names. Disregarding stereochemistry increases the consistency (84.8% to 99.9%). A wide variation in consistency also exists between MOL representations of compounds linked via cross-references (25.8% to 93.7%). Removing stereochemistry improved the consistency (47.6% to 95.6%).
Conclusions:
We have shown that considerable inconsistency exists in structural representation and systematic chemical identifiers within and between databases. This can have a great influence especially when merging data and if systematic identifiers are used as a key index for structure integration or cross-querying several databases. Regenerating systematic identifiers starting from their MOL representation and applying well-defined and documented chemistry standardisation rules to all compounds prior to creating them can dramatically increase internal consistency.
Abstract
Background:
There are two line notations of chemical structures that have established themselves in the field: the SMILES string and the InChI string. The InChI aims to provide a unique, or canonical, identifier for chemical structures, while SMILES strings are widely used for storage and interchange of chemical structures, but no standard exists to generate a canonical SMILES string.
Results:
I describe how to use the InChI canonicalisation to derive a canonical SMILES string in a straightforward way, either incorporating the InChI normalisations (Inchified SMILES) or not (Universal SMILES). This is the first description of a method to generate canonical SMILES that takes stereochemistry into account. When tested on the 1.1 m compounds in the ChEMBL database, and a 1 m compound subset of the PubChem Substance database, no canonicalisation failures were found with Inchified SMILES. Using Universal SMILES, 99.79% of the ChEMBL database was canonicalised successfully and 99.77% of the PubChem subset.
Conclusions:
The InChI canonicalisation algorithm can successfully be used as the basis for a common standard for canonical SMILES. While challenges remain – such as the development of a standard aromatic model for SMILES – the ability to create the same SMILES using different toolkits will mean that for the first time it will be possible to easily compare the chemical models used by different toolkits.